Kerry Weinberg:
Where we are with biology is where we were with aerospace in the early part of the 20th century. We’re really at the beginning. The power of machine learning is that we can better understand biology, and that will ultimately make it much faster and more efficient for us to get products to market.
Kerry Weinberg’s diverse career has spanned biology, computer science, teaching, and business administration. Now head of data science for Digital Health and Innovation at Amgen in Thousand Oaks, CA, she is working on artificial intelligence (AI) applications. In this interview, she reflects on her path and offers advice to students interested in the burgeoning field.
Can you tell us a bit about your high school experience and what got you on the path you are on now?
I went to Stuyvesant High School in New York City, which is a science-based high school. I was really passionate about math and science, and I worked at a lab at Cornell Medical College throughout high school. I was working in developmental biology and remember wondering why it was me as a scientist making the decision on whether a cell was different or not. Why not use computers to do that? And that was what got me initially interested in computer science Stuyvesant was great with getting me exposed to computer science even as a sophomore in high school. I was extremely fortunate to get the opportunity to work in a lab in high school, and that ultimately led me to biological engineering at MIT.
So, you went from high school to MIT, then what?
I'll be frank: I didn't really know what I wanted to do after graduation--really until senior year. I worked in a couple different labs during summers. I taught swimming to kids in New York public pools. One January break, I taught middle school math in South Bronx. I applied for Teach for America but didn't get in. I'm glad that things worked out the way they did because I wouldn't be where I am now if not for that; sometimes, rejections are good. After graduating, I was lucky to get a fantastic engineering rotational program job at Beckman Coulter, a company that develops and manufactures instruments that you use in the lab. I was at Beckman for about four years.
What was that job like?
I worked in Ft. Collins, Colorado, which is really different from where I’m from and where I went to school. It was a great experience. I worked with a small group of engineers on high-speed cell sorters, which sort cells at 100,000 cells per second. They are frequently used in academic laboratories at universities across the globe. I got really interested in operations. I debated during my time at Beckman whether I wanted to go back to grad school and get my Ph.D or not. Ultimately, I decided to prioritize understanding how business decisions are made. That led me to go back to MIT for a dual-degree program where I got my MBA from Sloan and my master’s in biological engineering.
So how did you end up at Amgen?
During my time in the dual-degree program, which is called Leaders for Global Operations, I did my master’s thesis research at Amgen, studying genetic drivers behind high-productivity cell lines. Amgen engineers cells to produce different proteins, so it’s really important to understand why certain cells are more productive than others. I then joined Amgen in 2016 in a digital integration role in process development. Ultimately, that led me into a role leading AI strategy across process development, which then led me last year into leading data science for digital health and innovation at Amgen.
What’s your typical day like at work?
It’s pretty varied, but most of my time is spent interacting with people. I lead a team of data scientists. Most of my time is spent helping them out with different projects that we’re working on and working with other people, like scientists, clinicians, marketers, and engineers across the company, to better understand important problems we should be solving. We’ll have calls with external folks quite a bit, like with startups and researchers. I’m an extrovert, so I love it.
What advice do you give to new team members just starting off?
I tell them they’re a lot smarter than they sometimes give themselves credit for. I try to give them accountability so that they are more in charge of their own destiny. It’s a big shift because in school, you are often told: This is the project, this is what you have to do, this is when it’s done. And your teacher is constantly on top of you. But in real-world work, you have deadlines, but no one’s going to tell you how to do it; you have to figure it out. So, I try to ease them into that a bit.
What do you see as the trends in AI right now?
I think we’re only going to see it being used more in healthcare. We’re beginning to have a more realistic impression of what is required. In the past two years, the question was: Is AI even applicable? We very quickly answered, “yes.” We have tried machine learning in a variety of use cases and found tremendous feasibility. So, it’s no longer a question of if it works; it’s when can we apply it and how fast? What will become continually challenging is making sure that we’re setting the right expectations.
What is the major challenge in the field right now?
One thing that is challenging for me right now is the lack of understanding of AI and machine learning has led to a proliferation of misuse of buzzwords, and, also on the opposite side, almost a disregard for mathematical techniques that may not be as sexy but are equally or more effective.
So how do you define AI in your work?
I primarily define machine learning as a technique that is used where you have a computer algorithm that learns the relationship between inputs and outputs. I have an input and an output, and my machine is figuring out what representation relates them the best. AI is when something that could be done by a human is done by a machine. And you could think of very routine tasks that humans do, but it’s typically thought of as human decision-making instead done by something artificial. Machine learning is exciting because having an algorithm help you understand the relationship between the inputs and outputs is particularly valuable for biology. We have so many pieces of data that we’re collecting, from genomics to protein levels to response to therapies, that we need the algorithms to give us more information. Where we are with biology is where we were with aerospace in the early part of the 20th century. We’re really at the beginning. The power of machine learning is that we can better understand biology, and that will ultimately make it much faster and more efficient for us to get products to market.
What advice do you have for students interested in data science?
Many algorithms are not point-and-click solutions. You actually have to know how to work with computers and build computer programs. So, I typically recommend people take a computer programming class. It is never too early to learn how to code. I taught 8-year-olds how to code. It was a very different language called Alice, a visual programming language developed by Carnegie Mellon that is a way to teach the concepts of programming and it was intended to increase female participation. And 8- year-olds were doing it and they were teaching their parents. I strongly believe there is no wrong age to start coding. My dad is 72, and he got a foot fracture recently. And he was so bored, and I said, “why don’t you teach yourself Python?” So, he started to teach himself Python. In general I believe that if you’re passionate about it, you should just do it.
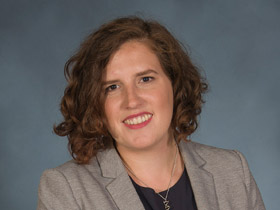