This story is the first part of an ongoing ABE series on data science in biotechnology.
Every human cell is packed full of proteins twisted and folded into specific shapes. The shapes of proteins help determine a protein’s function, making them crucial for discovering how diseases work and developing new drugs. In July 2021, a technology company called DeepMind announced it had used artificial intelligence (AI) to predict the shape of almost every protein in the human body, as well as hundreds of thousands of proteins in other organisms. Although the accuracy varied, they were able to share some 350,000 total newly predicted protein structures.
This development is just one of many recent breakthroughs in biotechnology, made possible by a large and growing body of biological data combined with advances in computational power. The explosive growth of biological data over the past decade is allowing scientists to perform a wide range of experiments much more rapidly and inexpensively than historically possible, even just 20 years ago. And indeed, we have seen the dramatic effect of these data throughout the COVID-19 pandemic, influencing everything from the speed of vaccine development to the tracking of symptoms and side effects—with information technology helping to provide new tools in identifying local outbreaks as well as guiding regulatory decisions on rare vaccine side effects.
From Bioinformatics to Omics to Deep Learning
Traditionally, the convergence of biology and computer science has been known as “bioinformatics,” focusing on the sequencing of proteins in the early 1950s and then DNA in the 1970s. The role of bioinformatics is to analyze and understand such large and complex biological data sets, covering everything from genomics (study of genomes) and transcriptomics (study of RNA transcripts) to proteomics (study of proteins) and metabolomics (study of metabolites), among others.
The study of these data, collectively referred to as “omics,” is invaluable to biotechnologists working to diagnose, prevent, and treat human diseases. Researchers can take the data from these different areas to better understand and treat diseases.
Indeed, pharmaceutical companies use biological data across their research and development processes in a number of ways: to find new drug targets, predict drug safety, assess previously published research, better understand individual differences in disease response, identify patients at higher risk, improve clinical trial design, and accelerate drug development.
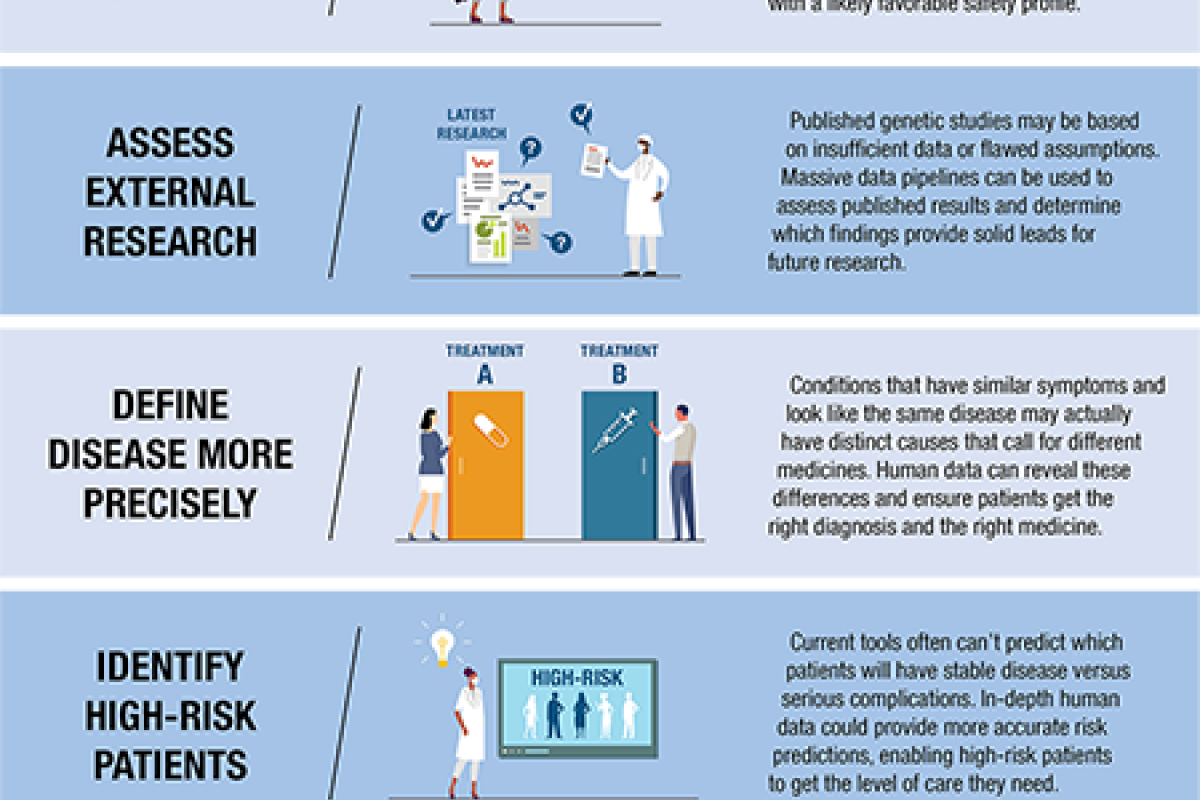
As the amount of biological data has grown, so have the tools used to understand and analyze it. Whereas biologists used to only have slow computers, early sequencers, and microscopes, modern biologists have high-powered supercomputers, rapid sequencing technology, and machine learning tools at their disposal.
Machine learning is a subset of AI that uses algorithms to quickly perform data analysis that was previously done by hand or was simply not possible due to the complex relationships between various groups of data. Combined with high-powered computing, machine learning has enabled “deep learning” breakthroughs such as the recent DeepMind protein shape predictions. The same set of tools also allow researchers to test libraries of known drugs against potential disease targets and to identify genetic and other risk factors for disease, supercharging drug discovery processes.
Beyond Drug Development
Data science is not only transforming drug development; it is also changing the way researchers can understand, diagnose, and track diseases. In 2020, Amgen’s subsidiary deCode Genetics tracked the spread of COVID-19 in Iceland by studying genetic mutations in the SARS-CoV-2 virus and measuring the durability of human antibodies to the virus.
But biological data was also at play during the pandemic in more visible ways, such as apps to track COVID-19 symptoms and wearables to track baseline vitals. Indeed, wearable technology such as Fitbits are contributing to the body of human health data that can be analyzed to help develop new biotechnology.
Such wearables are also allowing biopharmaceutical companies to monitor patients and track drug side effects in new ways. For example, in a new trial at Amgen, researchers are testing the use of wearable technology in monitoring leukemia patients at home, potentially helping to reduce prolonged hospital stays.
Other researchers are using deep learning to help radiologists interpret medical imaging results. For example, machine learning has the potential to use brain scanning results to identify a certain type of stroke more quickly than a trained technician could. Studies are ongoing to assess the best use cases for this type of machine-assisted diagnostics.
As the applications for biological data widen, the opportunities are also expanding for new career paths in data science within biotechnology. In the next stories in this series, ABE will profile some of the exceptional people working at the intersection of biotechnology and data science. ###
For more on our data science series, read Bridging the Gap Between Immunology and Data Science.